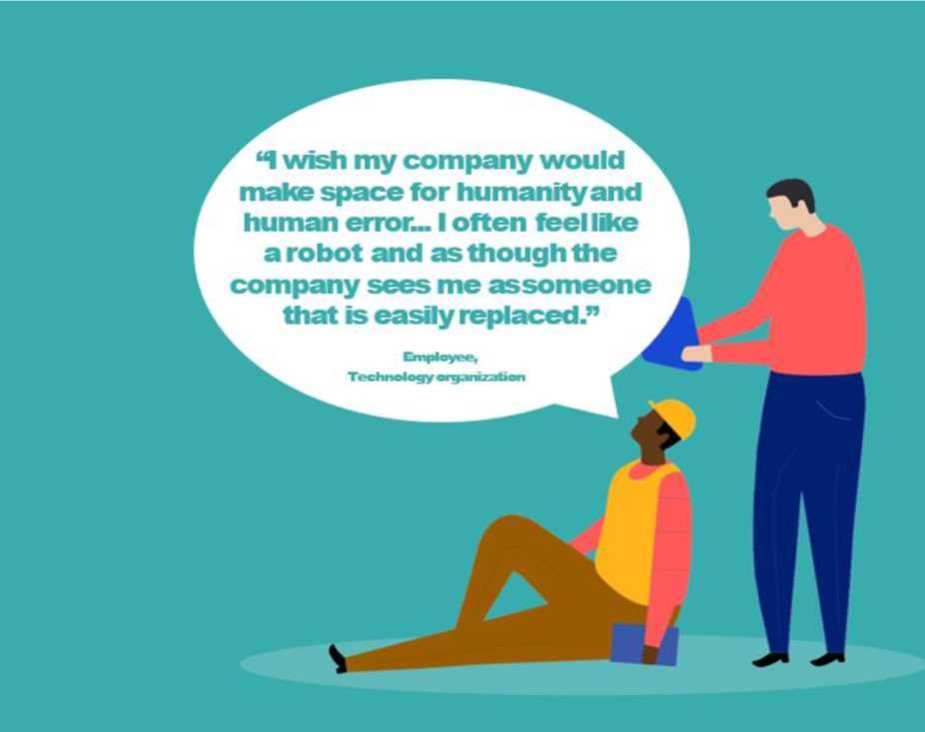
You've probably heard about deep learning for regression. Deep learning for regression can be a powerful new technology. It can predict the weather and even find out what your children eat for breakfast. But what does it mean for regression? Let's take a look at some of the key principles behind deep learning for regression. It is important to note that deep learning can be used in many different ways. There are two methods that can be used to achieve deep learning.
Less-squares regression
There are two types. One is mathematically easy, which places restrictions on input data. The other is mathematically more complex. Although the former offers the benefit of learning from a smaller training set, it is harder to use and detect errors. As a result, simpler procedures should be used whenever possible. Here are some examples of least-squares regression procedures.
Also known as the Residual Sum Squares, Ordinary least squares can also be called the Residual Sum Squares. It is a kind of optimization algorithm in which an initial cost function is used to increase or decrease the parameters until a minimum is reached. Important to note is that this method assumes that there is no sampling error distribution. It can still be used even if there is a deviation from the normal distribution of samples. This is a common limitation with least-squares.
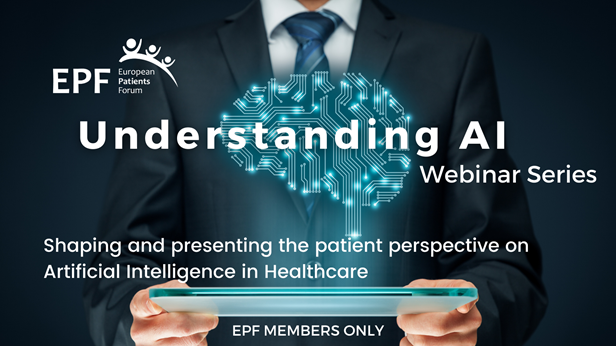
Logistic regression
Logistic analysis is a statistical method that predicts the likelihood of a particular outcome based in data science. Logistic regression, like other supervised machine-learning models, is useful in predicting trends. It classifies inputs into either a binary or multinomial group. For example, a binary logistic regression model can help identify high-risk individuals who are at higher risk of developing cancer than someone with low-risk status.
This technique is used to predict whether a person will pass a test or fail it based on the score. If a student studies for just one hour per week, they could score 500 higher than someone who studies for three hours each day. In this case, the probability that the student will pass the test would be zero if he or she had studied for three hour a day. However, logistic regression does not provide the same accuracy.
Support vector machines
SVMs, or support vector machines, are widely used in statistical machine learning. These algorithms are based upon a kernel-based method. This allows them to be flexible, adaptable, and versatile. This is important in certain types of applications. This article will discuss the advantages of using SVMs for regression. This article will discuss some of the key characteristics of these models. To understand how they work, let us start with some of the most common examples.
Support vector machines are highly effective on datasets with many features. Unlike other types of machine learning, these models require only a small set of training points. These models are memory efficient because they can use many different kernel functions. Additionally, you can specify the decision function either as custom or common. Avoiding over-fitting is the most important thing to remember when choosing the kernel function. SVMs are best suited for small sample sets and require extensive training.
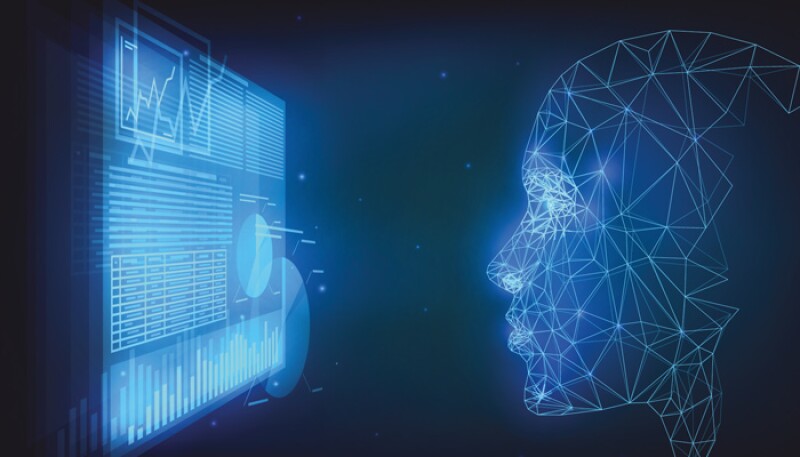
KNN
KNN is often called instance-based, lazy or lazy learning. This algorithm doesn't need to know the form of the problem or make assumptions about its data. Because of this, it is suitable for classification and regression problems. KNN's algorithm is extremely versatile and can easily be applied to real-world datasets. It is however slow and inefficient in fast prediction environments.
KNN algorithms use a number of examples in close proximity to predict a numerical value using data. For example, it can be used to rate the quality of a film by combining the values of k examples. The K value is usually averaged from the neighbors. However, the algorithm can also use weighted median or average. Once trained, the KNN algorithm may be used to predict from thousands upon thousands of images.
FAQ
What are some examples of AI applications?
AI is used in many areas, including finance, healthcare, manufacturing, transportation, energy, education, government, law enforcement, and defense. These are just a few of the many examples.
-
Finance – AI is already helping banks detect fraud. AI can detect suspicious activity in millions of transactions each day by scanning them.
-
Healthcare – AI is used for diagnosing diseases, spotting cancerous cells, as well as recommending treatments.
-
Manufacturing - AI in factories is used to increase efficiency, and decrease costs.
-
Transportation - Self Driving Cars have been successfully demonstrated in California. They are being tested in various parts of the world.
-
Energy - AI is being used by utilities to monitor power usage patterns.
-
Education - AI has been used for educational purposes. Students can interact with robots by using their smartphones.
-
Government – Artificial intelligence is being used within the government to track terrorists and criminals.
-
Law Enforcement - AI is used in police investigations. Search databases that contain thousands of hours worth of CCTV footage can be searched by detectives.
-
Defense - AI systems can be used offensively as well defensively. Offensively, AI systems can be used to hack into enemy computers. In defense, AI systems can be used to defend military bases from cyberattacks.
Why is AI used?
Artificial intelligence refers to computer science which deals with the simulation intelligent behavior for practical purposes such as robotics, natural-language processing, game play, and so forth.
AI is also called machine learning. Machine learning is the study on how machines learn from their environment without any explicitly programmed rules.
Two main reasons AI is used are:
-
To make your life easier.
-
To be better than ourselves at doing things.
Self-driving automobiles are an excellent example. AI can do the driving for you. We no longer need to hire someone to drive us around.
What is the newest AI invention?
Deep Learning is the most recent AI invention. Deep learning, a form of artificial intelligence, uses neural networks (a type machine learning) for tasks like image recognition, speech recognition and language translation. Google was the first to develop it.
Google recently used deep learning to create an algorithm that can write its code. This was done with "Google Brain", a neural system that was trained using massive amounts of data taken from YouTube videos.
This enabled the system learn to write its own programs.
IBM announced in 2015 they had created a computer program that could create music. Music creation is also performed using neural networks. These are known as NNFM, or "neural music networks".
Why is AI important
It is expected that there will be billions of connected devices within the next 30 years. These devices will include everything, from fridges to cars. The Internet of Things is made up of billions of connected devices and the internet. IoT devices will be able to communicate and share information with each other. They will be able make their own decisions. Based on past consumption patterns, a fridge could decide whether to order milk.
It is estimated that 50 billion IoT devices will exist by 2025. This represents a huge opportunity for businesses. This presents a huge opportunity for businesses, but it also raises security and privacy concerns.
What's the status of the AI Industry?
The AI market is growing at an unparalleled rate. By 2020, there will be more than 50 billion connected devices to the internet. This will enable us to all access AI technology through our smartphones, tablets and laptops.
This means that businesses must adapt to the changing market in order stay competitive. Companies that don't adapt to this shift risk losing customers.
It is up to you to decide what type of business model you would use in order take advantage of these potential opportunities. What if people uploaded their data to a platform and were able to connect with other users? You might also offer services such as voice recognition or image recognition.
Whatever you decide to do in life, you should think carefully about how it could affect your competitive position. Although you might not always win, if you are smart and continue to innovate, you could win big!
Where did AI originate?
Artificial intelligence was established in 1950 when Alan Turing proposed a test for intelligent computers. He stated that a machine should be able to fool an individual into believing it is talking with another person.
John McCarthy wrote an essay called "Can Machines Thinking?". He later took up this idea. In 1956, McCarthy wrote an essay titled "Can Machines Think?" He described the difficulties faced by AI researchers and offered some solutions.
Statistics
- In the first half of 2017, the company discovered and banned 300,000 terrorist-linked accounts, 95 percent of which were found by non-human, artificially intelligent machines. (builtin.com)
- A 2021 Pew Research survey revealed that 37 percent of respondents who are more concerned than excited about AI had concerns including job loss, privacy, and AI's potential to “surpass human skills.” (builtin.com)
- While all of it is still what seems like a far way off, the future of this technology presents a Catch-22, able to solve the world's problems and likely to power all the A.I. systems on earth, but also incredibly dangerous in the wrong hands. (forbes.com)
- Additionally, keeping in mind the current crisis, the AI is designed in a manner where it reduces the carbon footprint by 20-40%. (analyticsinsight.net)
- More than 70 percent of users claim they book trips on their phones, review travel tips, and research local landmarks and restaurants. (builtin.com)
External Links
How To
How do I start using AI?
Artificial intelligence can be used to create algorithms that learn from their mistakes. This allows you to learn from your mistakes and improve your future decisions.
A feature that suggests words for completing a sentence could be added to a text messaging system. It would learn from past messages and suggest similar phrases for you to choose from.
It would be necessary to train the system before it can write anything.
Chatbots can also be created for answering your questions. For example, you might ask, "what time does my flight leave?" The bot will reply that "the next one leaves around 8 am."
Our guide will show you how to get started in machine learning.